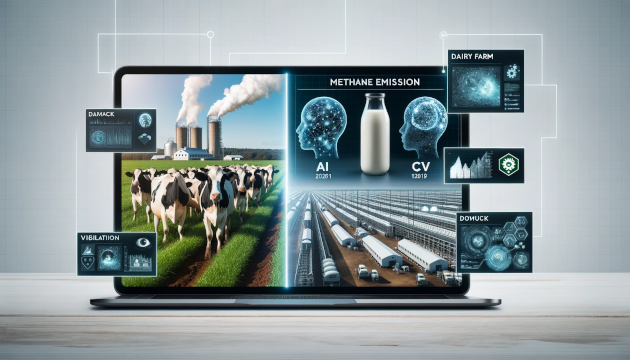
USDA grants awarded to Dr. Khaled Ahmed
Artificial Intelligence (AI) and computer vison (CV) have incredible potential in developing myriad of applications for various sectors such as agriculture. A motivation for the multidiscipline joint-research in AI and animal science is due to our passion to promote recent and future technologies for food and agriculture research and farming societies. Accordingly, we received awards for two multidiscipline projects with total $300K from USDA. This first project titled “Artificial Intelligence for Greener Livestock: Educational and Research”. It includes education and research approaches to explore the use of AI for greener livestock. The education part includes two activities to organize educational workshops to: 1) incorporate the artificial intelligence (AI) in the animal sciences for animal science students, and 2) disseminate the recent and future AI techniques in the animal sciences to the relevant societies. The multidiscipline research component addresses an animal science challenge; enteric methane emission in livestock. Methane is one of the greenhouse gases (GHG) that contributes to global warming. Enteric methane is the single largest source of direct GHG emissions in livestock sector, representing 2.5 percent of total US GHG. To promote greener livestock, addressing challenging tasks such as detecting, monitoring and recording enteric methane emissions from cows on large scale farms under different diets and farming conditions are essential. In this project, AI and CV will be used to promote greener livestock through improving methane production measurement and monitoring. Therefore, this project will 1) use gas infrared camera (IR) to collect two datasets of digital images of enteric methane emission under in vitro and in vivo conditions and 2) develop deep convolution neural networks (CNN) to generate deep methane measurements models (Deep3M). These models will be used to develop low-cost solutions that can measure enteric methane emission in real-time.
The second project titled “Detecting Subacute Ruminal Acidosis Using A Real-Time Deep Learning Algorithm”. It addresses an animal science challenge; Sub-acute ruminal acidosis (SARA). SARA is a common metabolic disease in an intensive cattle farm production system. Cattle with SARA experience significant drop in their performance (e.g. feed intake, milk production and weight gain), health (laminitis, organs inflammation, and tissues damages) and welfare. It has been estimated that the annual losses in the U.S. dairy industry due to SARA is $1 Billion. The early detection of SARA is important as it would allow cattle and dairy producers to correct the cause(s) for this metabolic disease (e.g. ration composition, soring, slug feedings, etc.) and therefore minimize the disease impact on animals. The current techniques used to early detect SARA are traditional and most of them have several limitations (e.g. accuracy and applicability) that limit their use under a traditional production system. In this project, AI and CV will be used to help in developing models that are capable to early detect SARA using the gasses composition naturally emitted by ruminant animals. Therefore, this project will 1) use gas infrared cameras (IR) to collect datasets of digital images of emitted gasses (CO2 and CH4) under different rumen pH conditions using an in vitro system as a model for ruminant animals, and 2) develop deep convolution neural networks (CNN) to generate deep SARA detection models (saraDeepG2M). These models will be used to develop low-cost solutions that can early detect SARA in real-time. The education part includes two activities to organize educational workshops to: 1) incorporate the AI in the animal sciences for animal science students, and 2) disseminate the recent and future AI techniques in the animal sciences to the relevant societies.
The expected results from these projects will provide practical solutions for animal nutrition research that are time effective, low cost, more precise and environmentally friendly.